Wind energy: Increasing yield with IoT, Digital Twins and AI
Wind power is a cornerstone of sustainable energy generation in many countries, and increasing its yields is therefore of great importance. In the WindIO research project, CONTACT Software and its partners have developed an industry-specific IoT solution that significantly improves the wind turbines’ efficiency.
The Digital Twins of a Krogmann and a Senvion wind turbine are represented in a test instance of the CONTACT Elements for IoT platform. These turbines are made available for research purposes to the WindIO project under the leadership of the University of Bremen.
Digital Twin as a key technology
The industry has not fully exploited the potential that Digital Twins of wind turbines offer. A Digital Twin represents the operational state of the turbine, manages environmental data such as wind speed and direction, and supports the prediction of suitable maintenance windows and expected energy yields.
The Krogmann turbine is equipped with LiDAR, sensors in the rotor blades, and a sensor box. These measure current environmental parameters such as temperatures, tower vibration (through acceleration sensors in the sensor box), and local weather data like wind speeds at different altitudes. The data is transmitted to the IoT platform via the standard protocol MQTT, analyzed by algorithms, and visualized in the dashboards for the operations management of the plant.
Dashboards for operations management
The IoT platform supports the technical operations management of the wind turbines through specialized dashboards. They enable continuous condition monitoring, provide information about weather conditions, and streamline the planning and execution of maintenance.
The maintenance dashboard provides a calendar that displays and signals upcoming work. This applies to both scheduled maintenance and unplanned repairs resulting from a malfunction. Preconfigured KPIs (Key Performance Indicators) are available on the dashboard to track operating hours, downtime, and the number of events. In addition, there are overview tables for executed service jobs, whose details, such as installed spare parts and required working hours, can be viewed directly via a link. Incidents at the turbine, such as malfunctions or unplanned downtimes and their causes, are also documented in the overview tables.
AI for the optimal time window
The maintenance of wind turbines requires favorable weather conditions. The data from the sensor box on the tower of the Krogmann plant makes it possible to predict the optimal time window for blade maintenance [1]. Within the WindIO project, the partners have implemented a model based on Machine Learning (ML) into the IoT platform. It calculates short-term maintenance forecasts from the tower's vibration measurement and local weather data. Depending on whether the conditions are suitable for a service or not, a traffic light on the corresponding dashboard switches to green or red.
The research team developed the prediction model using the SIMWORX solution from CONTACT's subsidiary CAIQ, which was integrated into Elements for IoT as part of the project. The ML model is programmed in Python. Plant manufacturers or operators can use a configurable template to automate the forecast calculation for the optimal maintenance window.
The elements necessary for the configuration, such as time series data from the Digital Twin of the respective turbine (asset), are easily placed into the template via drag and drop. Once the configuration is completed, the automation starts, and the template calculates suitable time windows. This also automates triggering new events, such as switching the dashboard traffic light.
Prediction of the energy yield
When it comes to renewable energies, it is crucial to forecast their energy yield to ensure the stability of the power grid. This is why Germany's National Meteorological Service DWD also participates in research projects to improve weather prediction models [2]. The WindIO team utilizes global weather data and local weather data recorded with the Krogmann turbine LiDAR to determine the site's local wind conditions. For this purpose, a prediction model based on decision trees is employed, which forecasts the expected energy yield using a simulation twin [3]. Again, the implementation was accomplished through the integration of SIMWORX into CONTACT Elements for IoT.
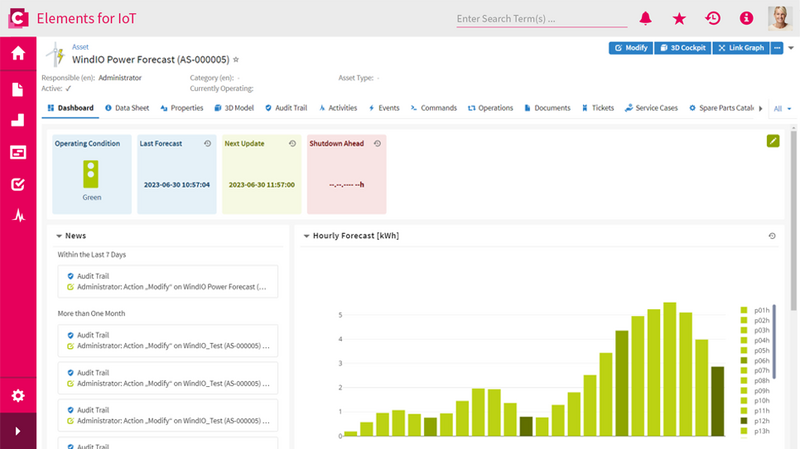
Sources
[1] Sander, A, Haselsteiner, AF, Barat, K, Janssen, M, Oelker, S, Ohlendorf, J, & Thoben, K. "Relative Motion During Single Blade Installation: Measurements from the North Sea." Proceedings of the ASME 2020 39th International Conference on Ocean, Offshore and Arctic Engineering. Volume 9: Ocean Renewable Energy. August 3–7, 2020. V009T09A069. ASME. https://doi.org/10.1115/OMAE2020-18935
[2] https://www.dwd.de/EN/research/weatherforecasting/weatherforecasting_node.html
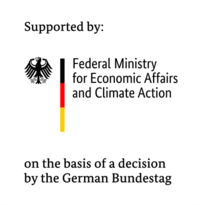