ASTRADIN
Automated flow-acoustic design optimization for the industry
The ASTRADIN project aimed at innovative software techniques for optimizing product acoustics. In contrast to costly prototype experiments, it relied on simulations using high-performance computers. The current aeroacoustic design process takes half a year and hundreds of work hours. Here, the project introduced self-learning methods based on deep neural networks for automated model creation, process monitoring, and improved result interpretation. Through clever automation and parallelization, product variations can be explored in less time.
- Project start: July 2019
- Runtime: 33 Months
- Founding amount: €1.2 million
- Number of partners: 4
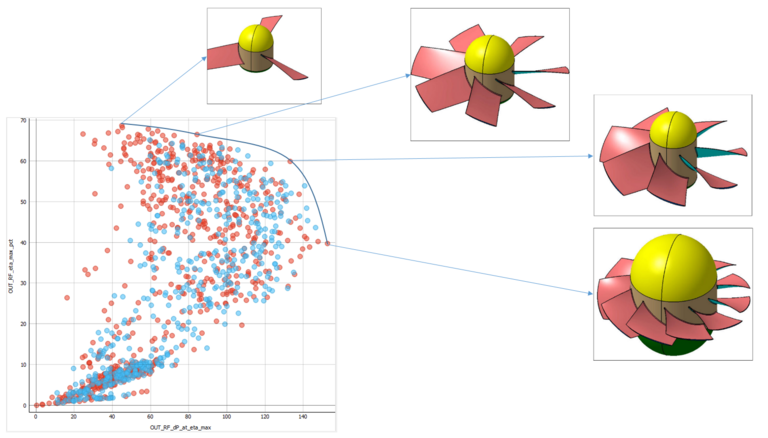
Objectives
For the first time, self-learning methods based on deep neural networks, especially Convolutional Neural Networks (CNNs), were explored as a novel foundation for necessary yet previously unavailable functions. The technologies for required software tools were developed accordingly.
The approach chosen in the ASTRADIN project and the resulting functionalities enabled an integrated, automated workflow that linked product simulations with optimization methods and Computer-Aided Design (CAD) data even in early development stages. This facilitated swift creation of virtual prototypes and simulated design variations, considering acoustic sources and sound wave propagation in detail.
The foundation rested on investigations into deep neural networks, particularly Convolutional Neural Networks (CNNs), for knowledge-based generation of structured networks for simulations, incorporation of knowledge-based decisions into the automatic monitoring and evaluation of simulations, and the creation of modular metamodels (quickly computable surrogate models) for accelerated optimization of parametric CAD models.
Through the ASTRADIN project, knowledge-based decisions could be integrated into the virtual prototyping process, enhancing its reliability and automating previously manual interactive steps that were previously only achievable through the extensive expertise of a simulation engineer.
The resulting software demonstrator of the project is based on the CONTACT Elements platform, utilizing it as the foundation for the "Optimization as a Service" approach, which was implemented alongside the application during the course of the project.
Objectives
For the first time, self-learning methods based on deep neural networks, especially Convolutional Neural Networks (CNNs), were explored as a novel foundation for necessary yet previously unavailable functions. The technologies for required software tools were developed accordingly.
The approach chosen in the ASTRADIN project and the resulting functionalities enabled an integrated, automated workflow that linked product simulations with optimization methods and Computer-Aided Design (CAD) data even in early development stages. This facilitated swift creation of virtual prototypes and simulated design variations, considering acoustic sources and sound wave propagation in detail.
The foundation rested on investigations into deep neural networks, particularly Convolutional Neural Networks (CNNs), for knowledge-based generation of structured networks for simulations, incorporation of knowledge-based decisions into the automatic monitoring and evaluation of simulations, and the creation of modular metamodels (quickly computable surrogate models) for accelerated optimization of parametric CAD models.
Through the ASTRADIN project, knowledge-based decisions could be integrated into the virtual prototyping process, enhancing its reliability and automating previously manual interactive steps that were previously only achievable through the extensive expertise of a simulation engineer.
The resulting software demonstrator of the project is based on the CONTACT Elements platform, utilizing it as the foundation for the "Optimization as a Service" approach, which was implemented alongside the application during the course of the project.
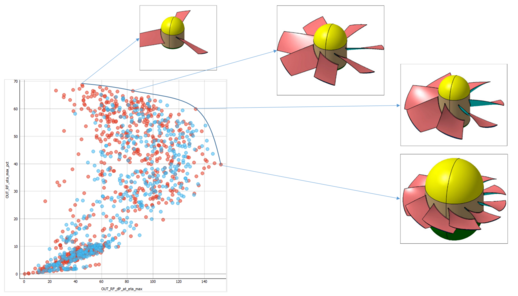
Objectives
For the first time, self-learning methods based on deep neural networks, especially Convolutional Neural Networks (CNNs), were explored as a novel foundation for necessary yet previously unavailable functions. The technologies for required software tools were developed accordingly.
The approach chosen in the ASTRADIN project and the resulting functionalities enabled an integrated, automated workflow that linked product simulations with optimization methods and Computer-Aided Design (CAD) data even in early development stages. This facilitated swift creation of virtual prototypes and simulated design variations, considering acoustic sources and sound wave propagation in detail.
The foundation rested on investigations into deep neural networks, particularly Convolutional Neural Networks (CNNs), for knowledge-based generation of structured networks for simulations, incorporation of knowledge-based decisions into the automatic monitoring and evaluation of simulations, and the creation of modular metamodels (quickly computable surrogate models) for accelerated optimization of parametric CAD models.
Through the ASTRADIN project, knowledge-based decisions could be integrated into the virtual prototyping process, enhancing its reliability and automating previously manual interactive steps that were previously only achievable through the extensive expertise of a simulation engineer.
The resulting software demonstrator of the project is based on the CONTACT Elements platform, utilizing it as the foundation for the "Optimization as a Service" approach, which was implemented alongside the application during the course of the project.
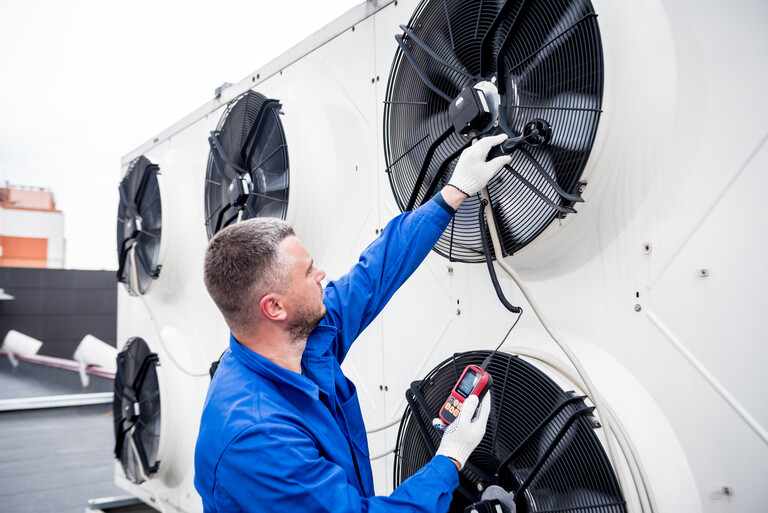
Motivation
The project aims to make the benefits of AI-based assistance systems accessible to industrial companies:
◾ Cost efficiency: By automating complex tasks with AI, human work hours and thus costs can be saved.
◾ Accelerated development: AI significantly shortens development times for components as it works faster and more efficiently than traditional methods. This results in faster product development cycles.
◾ Consistent quality: Thanks to its data-driven algorithms, AI consistently delivers precise results unaffected by human factors.
◾ User-friendliness: AI-based simulation applications are accessible even to non-experts, allowing individuals without deep expertise to make informed decisions and develop innovative solutions.
In summary, AI-based assistance systems enhance the efficiency, speed, and quality of the development process in a service-oriented product design and make the technology accessible to a broader range of users.
Motivation
The project aims to make the benefits of AI-based assistance systems accessible to industrial companies:
◾ Cost efficiency: By automating complex tasks with AI, human work hours and thus costs can be saved.
◾ Accelerated development: AI significantly shortens development times for components as it works faster and more efficiently than traditional methods. This results in faster product development cycles.
◾ Consistent quality: Thanks to its data-driven algorithms, AI consistently delivers precise results unaffected by human factors.
◾ User-friendliness: AI-based simulation applications are accessible even to non-experts, allowing individuals without deep expertise to make informed decisions and develop innovative solutions.
In summary, AI-based assistance systems enhance the efficiency, speed, and quality of the development process in a service-oriented product design and make the technology accessible to a broader range of users.
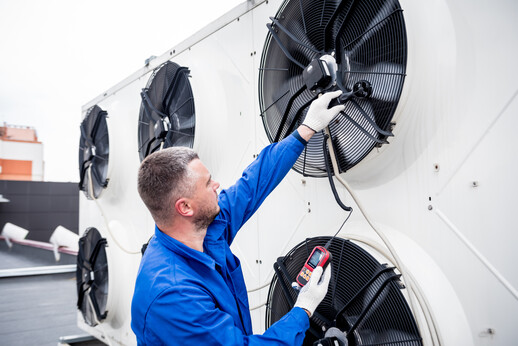
Motivation
The project aims to make the benefits of AI-based assistance systems accessible to industrial companies:
◾ Cost efficiency: By automating complex tasks with AI, human work hours and thus costs can be saved.
◾ Accelerated development: AI significantly shortens development times for components as it works faster and more efficiently than traditional methods. This results in faster product development cycles.
◾ Consistent quality: Thanks to its data-driven algorithms, AI consistently delivers precise results unaffected by human factors.
◾ User-friendliness: AI-based simulation applications are accessible even to non-experts, allowing individuals without deep expertise to make informed decisions and develop innovative solutions.
In summary, AI-based assistance systems enhance the efficiency, speed, and quality of the development process in a service-oriented product design and make the technology accessible to a broader range of users.
Project Partners
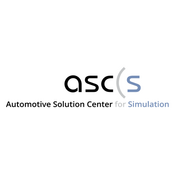
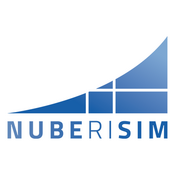
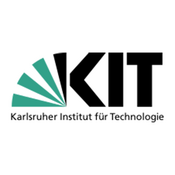
This research and development project was funded by the German Federal Ministry of Education and Research (BMBF) as part of the KMU-Innovativ program.
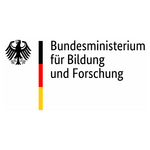
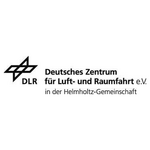